The Australian info TikTok owner ByteDance’s “Self-Controlled Memory system” can reach into a information bank of hundreds of turns of discussion, and thousands of characters, to offer any language design abilities remarkable to that of ChatGPT to response concerns about past occasions. ByteDanceWhen you type things into the timely of a generative synthetic intelligence (AI) program such as ChatGPT, the program provides you a reaction based not simply on what you’ve typed, however likewise all the things you’ve typed previously. You can believe of that chat history as a sort of memory. But it’s not enough, according to scientists at numerous organizations, who are attempting to enhance generative AI with something more like an arranged memory that can enhance what it produces. Also: How to usage ChatGPT: Everything you requirement to understand A paper released this month by scientist Weizhi Wang from University of California at Santa Barbara, and partners from Microsoft, entitled “Augmenting Language Models with Long-Term Memory”, and published on the arXiv pre-print server, includes a brand-new part to language designs. The issue is ChatGPT and comparable programs can’t take in sufficient text in any one minute to have a really long context for things. As Wang and group observe, “the input length limitation of existing LLMs avoids them from generalizing to real-world situations where the ability of processing long-form info beyond a fix-sized session is crucial.” OpenAI’s GPT-3, for example, takes optimum input of 2,000 tokens, significance, characters or words. You can’t feed the program a 5,000-word post, state, or a 70,000-word unique. Also: This brand-new innovation might blow away GPT-4 and whatever like it It’s possible to keep broadening the input “window,” however that runs into a tough computing issue. The attention operation — the important tool of all big language programs, consistingof ChatGPT and GPT-4 — has “quadratic” computational intricacy (see the “time intricacy” of calculating). That intricacy implies the quantity of time it takes for ChatGPT to produce an response increases as the square of the quantity of information it is fed as input. Increasing the window balloons the calculate required. And so some scholars, note Wang and group, have currently attempted to come up with a crude memory. Yuhuai Wu and associates at Google last year presented what they call the Memorizing Transformer, which shops a copy of previous responses that it can in future draw upon. That procedure lets it run on 65,000 tokens at a time. But Wang and group note the information can endupbeing “stale”. The procedure of training the Memory Transformer makes some things in memory endedupbeing out of sync with the neural network as its neural weights, or, criteria, are upgraded. Wang and group’s option, called “Language Models Augmented with Long-Term Memory”, or LongMem, utilizes a standard big language design that does 2 things. As it inspects input, it shops some of it in the memory bank. It likewise passes the output of every present timely to a 2nd neural network, called the SideNet. Also: How I fooled ChatGPT into informing me lies The SideNet, which is likewise a language design, simply like the veryfirst network, is charged with comparing the existing timely typed by a individual to the contents of memory to see if there’s a pertinent match. The SideNet, unlike the Memory Transformer, can be qualified on its own apart from the primary language design. That method, it gets muchbetter and muchbetter at selecting out contents of memory that won’t be stagnant. Wang and group run tests to compare LongMem to both the Memorizing Transformer and to OpenAI’s GPT-2 language design. They likewise compare LongMem to reported results from the literature for other language designs, consistingof the 175-billion criterion GPT-3. UC Santa Barbara, MicrosoftThey usage jobs based on 3 datasets that include summingup extremely long texts, consistingof whole shortarticles and books: Project Gutenberg, the arXiv file server, and ChapterBreak. To offer you an concept of the scale of those jobs, ChapterBreak, presented last year by Simeng Sun and associates at the University of Massachusetts Amherst, takes entire books and tests a language design to see if, provided one chapter as input, it can precisely recognize from numerous prospect passages which one is the start of the next chapter. Such a job “requires a abundant understanding of long-range dependences”, such as modifications in location and time of occasions, and methods consistingof “analepsis”, where, “the next chapter is a ‘flashback’ to an earlier point in the story.” Also: AI is more mostlikely to cause world doom than environment modification, according to an AI professional And it includes processing 10s or even hundreds of thousands of tokens. When Sun and group ran those ChapterBreak tests, they reported last year, the dominant language designs “struggled”. For example, the big GPT-3 was right just 28% of the time. But the Lon
Read More.
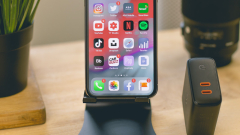